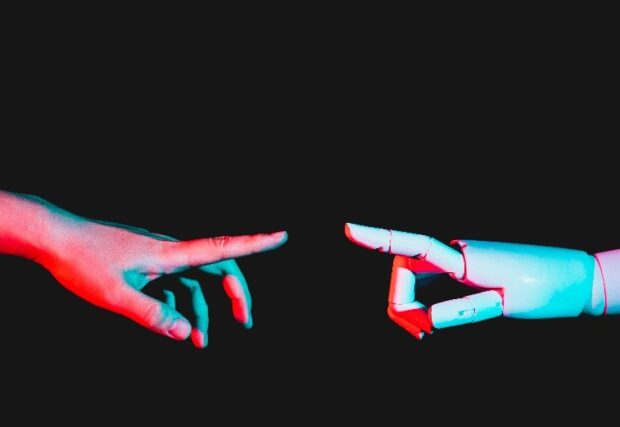
Natalie Chivite-Matthews
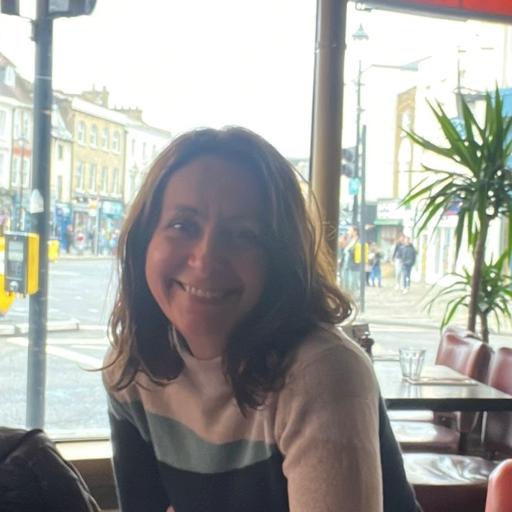
Arianna Rossi
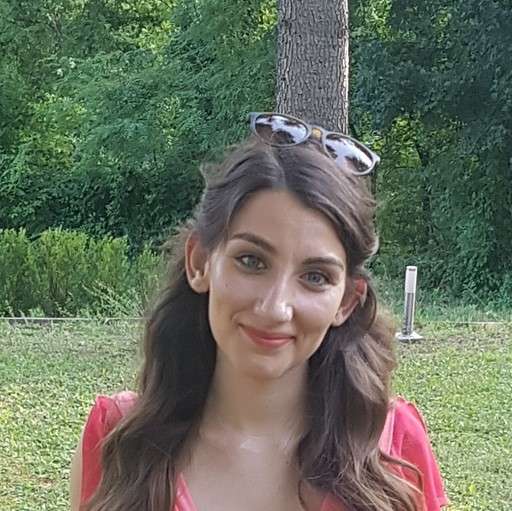
Giulia Torella
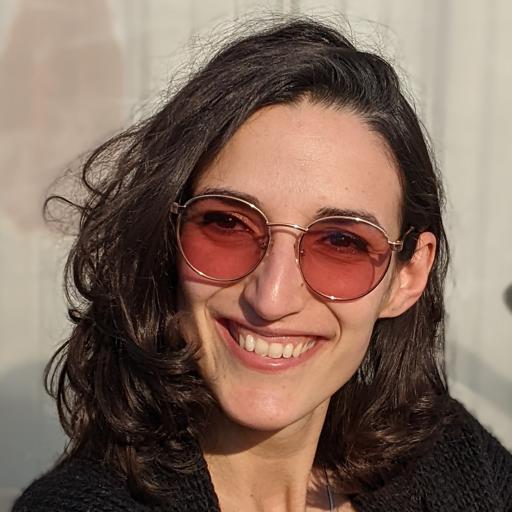
Karina Sacuiu
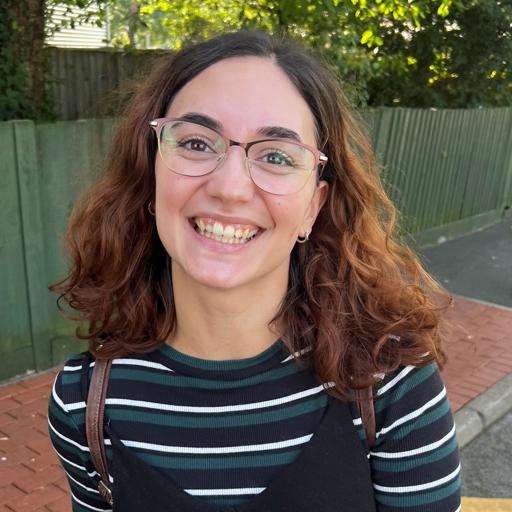
AI in Government
The UK Government launched an AI Opportunities Action Plan in early 2025 to accelerate AI adoption nationwide. Before that, the government has embarked on an ambitious journey to establish itself as a leader in Artificial Intelligence (AI) through its 2022 National AI Strategy. This plan aims to increase investment in innovation while fostering public trust in AI applications.
Generative Artificial Intelligence is a revolutionary branch of AI that not only mimics human intelligence but also seeks to surpass it. Although these developments have captured public interest, concerns remain about their effectiveness, risks and potential costs, especially in government applications.
As government departments start introducing a new type of AI by using Large Language Models (LLMs), it is important to:
- understand the life cycle of AI projects and the role of evaluation within them
- measure the performance, effectiveness, and value-for-money of these tools
Evaluation's role within an AI project
In DBT, we have a dedicated Digital Evaluation function where Social Researchers, Statisticians, Performance Analysts and Economists work together. They shine a light on the impact of our digital services, assess the processes behind them, and secure continuous improvement. We design and evaluate AI tools in accordance with specific guidance:
- the Green Book which refers to the design and use of Monitoring and Evaluation (M&E)
- the new Annex of the Magenta Book which covers best practice for evaluation of AI tools and technologies
M&E is involved in all stages of an AI project life cycle. This has been aligned with the government’s project cycle from the Green Book:
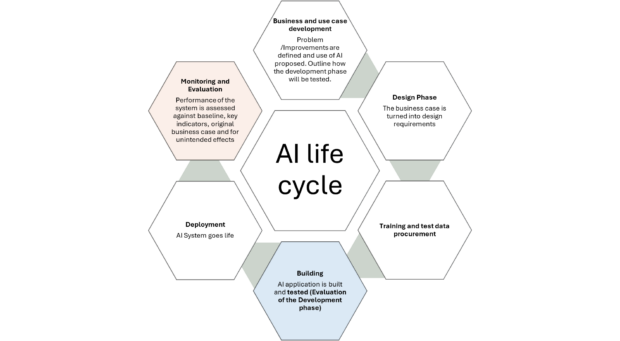
Evaluation is key for the development and deployment stages.
- Development Stage:
Evaluators identify the right performance indicators for this phase. Important factors include accuracy, speed, fairness, security, and the cost of errors. Testing at this stage is done by reflecting real-world use cases and evaluating models from multiple perspectives.
- Deployment Stage:
Evaluators focus on understanding the social and economic impacts of the AI tool. Critical questions include: Is the AI tool more effective than inaction? Can we approach this as an experiment? Can we identify a robust comparison group? Is it possible to compare with the existing delivery models? What is the error rate? What overall effects will it have on the population and are there any unforeseen consequences? What kind of monitoring will we need for this AI tool long term?
Robust M&E of AI systems are crucial for learning and accountability. For learning, evaluations provide valuable evidence that can help manage risks and uncertainties. They also inform decisions about whether to continue, improve, or discontinue an approach.
For accountability, evaluations shed light on the outcomes and value-for-money of government initiatives. They provide evidence of policy effectiveness used during Spending Reviews and in response to public scrutiny. The M&E of AI are also tracking whether the Government Digital Service (GDS) goals and principles are being met.
Evaluation techniques
Joe Justice
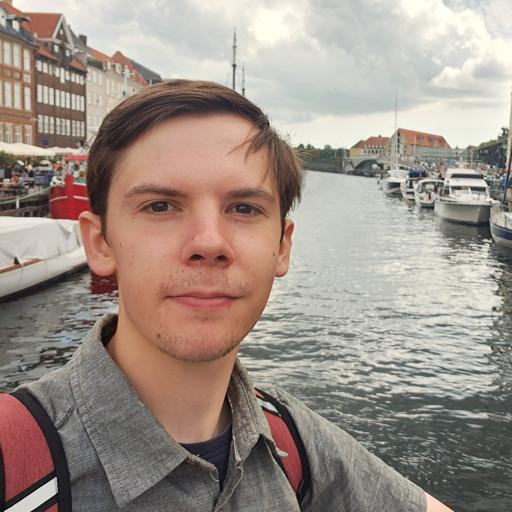
Wherever feasible in DBT, we apply multiple methods to evaluate the impact of AI interventions. A comprehensive evaluation may include:
- Monitoring: tracking data on key metrics before, during and after the intervention to help answer evaluation questions
- Process evaluation: analysing whether an intervention is being implemented as intended and what can be learned from how the intervention was delivered. These kinds of analysis are often used to assess iterative and evolving AI systems
- Impact evaluation: assessing the difference an intervention made by asking what measurable outcomes have occurred as a result of the intervention, whether intended or unintended. AI interventions are implemented rapidly, and impact evaluation needs to be embedded at all stages. Therefore collaboration between evaluators and development and delivery teams is crucial
- Value-for-money evaluation: determining whether the intervention and approach are a good use of resources, and if its benefits are outweighed by the associated costs
What this means for us
In the Digital Data and Technology (DDaT) M&E team in DBT, we are currently in the process of evaluating 2 AI tools: ‘M365 Copilot’ and the internally developed ‘Redbox’.
M365 Copilot
Daisy Thomas
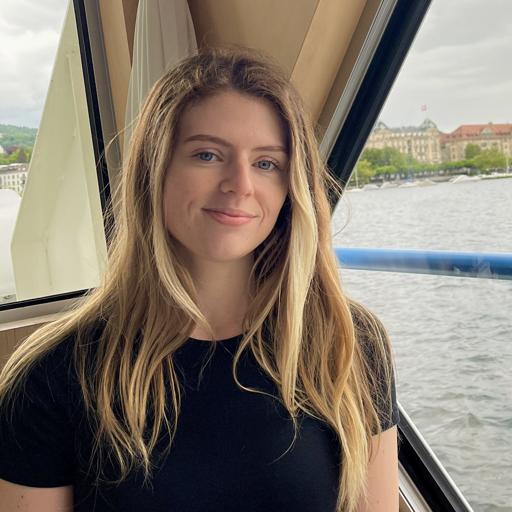
Between October and December 2024, a sample of M365 Copilot licences were distributed across DBT for a trial period. The results are currently being used to assess the impacts across the department, how and why people use the tool and for whom the risks or benefits are most noticeable.
To answer these questions, we are using multiple methods, with 4 main components:
- For a 1-week period in November, licence-holders completed a diary about experiences with the tool. The diaries provided data on what tasks were being performed and how much time was saved or lost. They also gave us feedback on the quality of outputs and how M365 Copilot has influenced ways of working.
- We will hold follow-up interviews with a diverse range of colleagues across the department. This will help us explore themes in greater depth and develop a better understanding of the benefits and challenges facing specific groups and individuals.
- To verify time-saving calculations and to better understand how M365 Copilot is used, we will run a series of testing exercises, observing colleagues completing the same task with and without M365 Copilot.
- Lastly, we will conduct a cost-benefit analysis to compare the tool's benefits against its costs (including to the environment) and risks to the Department, ensuring it provides value for money.
Redbox evaluation
Roseanna Morrall
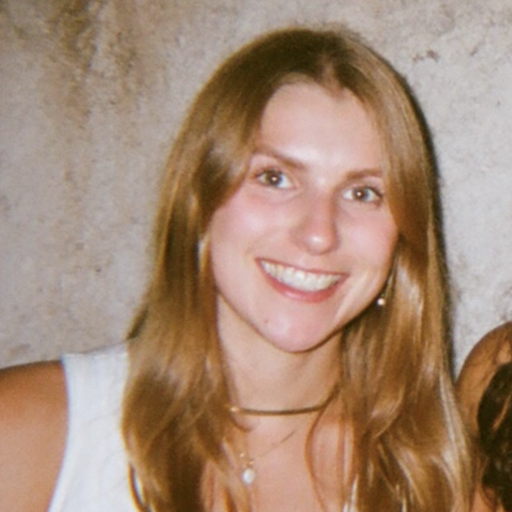
Redbox is an internal AI tool that was being developed by the Incubator of Artificial Intelligence (i.AI) from Cabinet Office to be used across government to develop public services. DBT have been developing this code base further, by building additional features sets into the tool to support departmental needs. This iteration of Redbox has been deployed to staff in DBT to trial until March 2025. Please check out this blog post on early outcomes from using Redbox in DBT.
The first evaluation phase is an exploratory one which involves the majority of Redbox users. It will deploy the following evaluation methods:
- A survey sent before and after users are given access to Redbox. This method is used to assess differences in how users undertake tasks before and after receiving access to Redbox. It will help us to understand if their expectations match the capabilities of this tool.
- A diary that the Redbox users will complete for a week. Similar to the M365 Copilot trial, diaries will be used to understand a range of use cases, how the tool affects productivity and whether any unforeseen issues arise.
The second phase will be comparative analysis, bringing M365 Copilot and Redbox evaluation findings together. It will include a sample of cross-over users with access to both Redbox and M365 Copilot, who will be assessed through both diaries and qualitative interviews. These methods will be used to understand how the capabilities of the 2 tools differ. They will also identify the use cases and user groups each tool delivers greatest value for in DBT. They will be supported by cost-effectiveness analysis.
Lessons learned
Our time working on the evaluations of M365 Copilot and Redbox has taught us some valuable lessons regarding the AI system evaluation including:
- engage stakeholders early and often: conversations with colleagues from various teams, professions and backgrounds introduced a broader range of perspectives to our evaluation, helping to identify potential risks, benefits and challenges beyond those we had initially considered
- approach AI systems flexibly and with an open mind: recognising the novelty of AI systems and the many unknowns surrounding them. It is important to continuously challenge pre-existing assumptions and remain open to changes or unexpected results
- combine multiple methods: combining quantitative data with qualitative insights helped us produce more well-rounded evaluations to illuminate what happened, why it happened and how different individuals experience these effects
- a consistent and co-ordinated communication plan: ensuring that colleagues understand what is being trialled and can confidently engage with new tools or technologies
Conclusion
AI tools hold the potential to transform our ways of working but equally pose a range of new challenges and uncertainties. By working collaboratively across disciplines and incorporating multiple methods into our approach, we hope to produce robust evaluations allowing DBT to confidently move forwards.
Read about the insights gained at DBT's first AI conference.
Leave a comment